Predicting Optimal Growth Conditions: Harnessing Machine Learning for Cannabis Cultivation
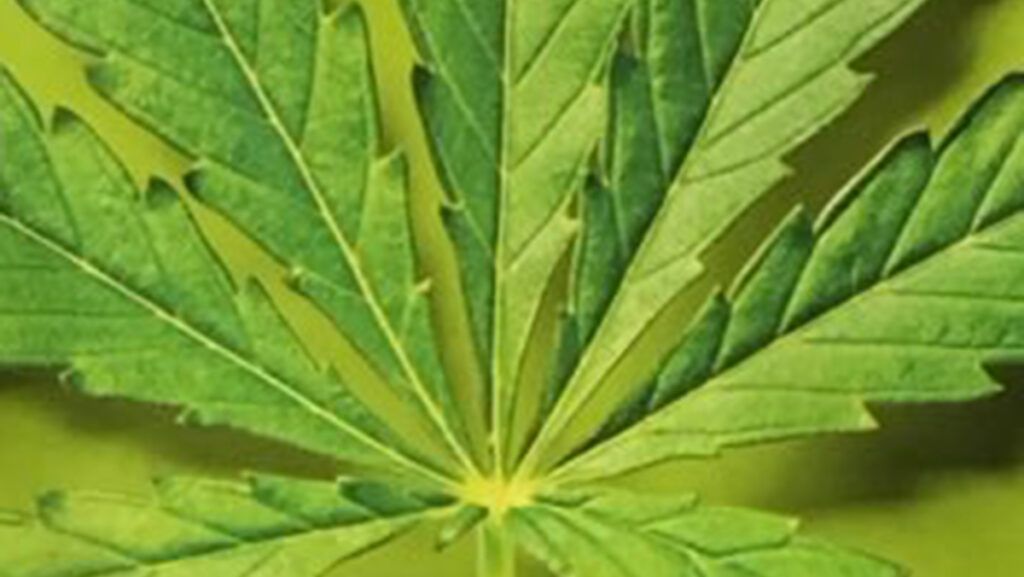
The cannabis industry is experiencing rapid growth, and with it comes the need for innovative approaches to cultivation. Among these, the use of machine learning (ML) to optimize growth conditions is revolutionizing how cultivators manage their crops. By analyzing vast datasets, ML models can predict the ideal light, temperature, humidity, and nutrient levels required for optimal cannabis growth, enabling cultivators to maximize yields, reduce waste, and enhance product quality.
One of the key advantages of ML in cultivation is its ability to process and interpret complex environmental interactions. For instance, light intensity and duration directly influence photosynthesis, but their effects can vary based on temperature and humidity. Traditional methods often rely on trial and error, which can be time-consuming and resource-intensive. ML algorithms, however, can identify patterns and correlations across multiple variables, offering precise recommendations tailored to specific cannabis strains.
Temperature and humidity play critical roles in the growth and health of cannabis plants. ML models can analyze historical climate data, real-time sensor readings, and plant health metrics to predict the optimal conditions for each growth stage. For example, during the vegetative stage, cannabis plants thrive in slightly warmer and more humid conditions, while the flowering stage requires cooler and drier environments. ML can fine-tune these parameters, ensuring plants receive exactly what they need at every stage.
Nutrient management is another area where ML is making significant strides. Cannabis plants require a delicate balance of nutrients, and deficiencies or excesses can lead to suboptimal growth or even plant failure. ML systems can monitor soil composition, pH levels, and plant health indicators to recommend precise nutrient formulations. Additionally, these models can adapt recommendations over time, accounting for changes in plant requirements as they grow and develop.
The integration of ML with advanced sensor technology further enhances cultivation optimization. Sensors provide real-time data on environmental conditions, which ML algorithms use to make continuous adjustments. This dynamic approach allows growers to respond to fluctuations in conditions promptly, maintaining an ideal growth environment. Furthermore, such systems can predict potential issues, such as pest infestations or mold outbreaks, by analyzing subtle changes in environmental or plant health data.
Leveraging ML for cultivation optimization is not just an advantage—it’s becoming a necessity. By predicting and maintaining ideal growth conditions, cultivators can achieve consistent, high-quality harvests while minimizing resource use and environmental impact. The future of cannabis cultivation lies in the hands of data-driven technology, and machine learning is leading the charge.